Citation link:
https://media.suub.uni-bremen.de/handle/elib/7553
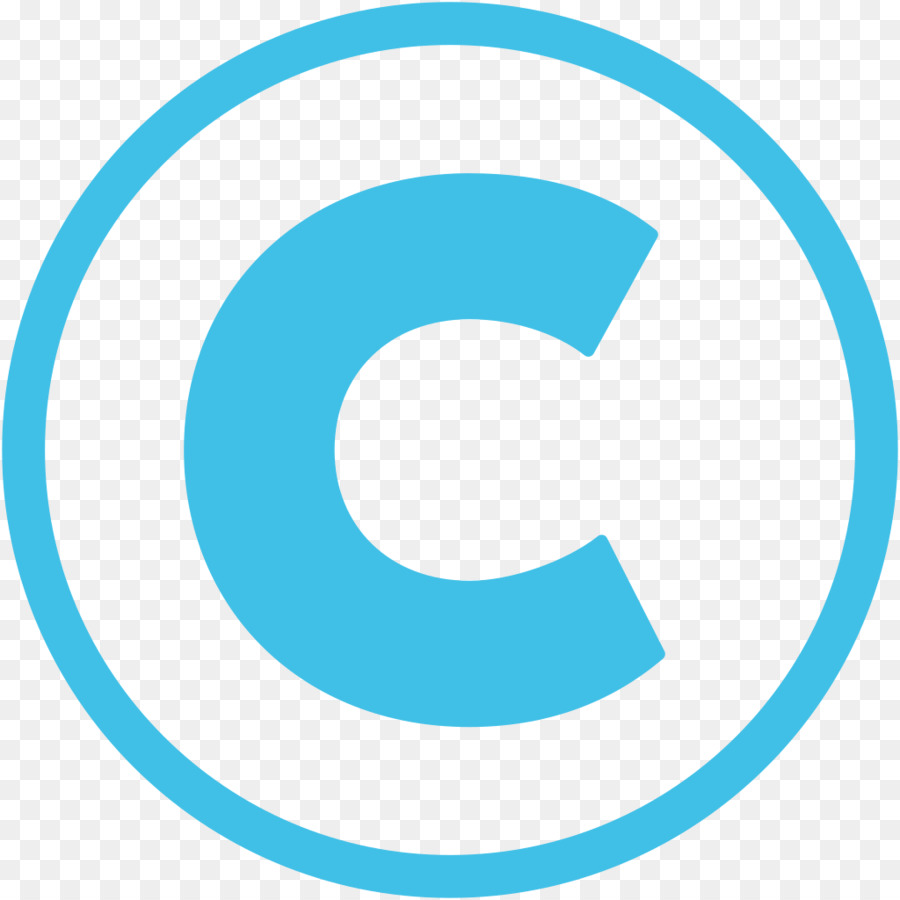
On the detection and classification of objects in scarce sidescan sonar image dataset with deep learning methods
Authors: | Steiniger, Yannik ![]() Stoppe, Jannis ![]() Kraus, Dieter ![]() Meisen, Tobias ![]() |
Abstract: | Applying deep learning detection methods to sonar imagery is a challenging task due to the complexity of the image itself as well as the limited amount of available data. In this work, we analyze one-step and two-step setups for detection multiple different objects in sidescan sonar images. The one-step setup and the first step in the two-step setup uses standard deep learning models, like YOLOv8, to either directly locate and classify the objects or to serve as a snippet extractor. In the second step these extracted snippets are further classified by a convolutional neural network. Furthermore, we investigate a setup in which the detected objects from the one-step approach are filtered by another CNN to reduce false alarms. Finally, we compare the performance of multiple deep learning detectors to a classical two-step approach using template matching combined with a CNN. Our results show that both two-step setups generate less false alarms. Furthermore, all deep learning models outperform the template matching approach. |
Keywords: | deep learning; Automatic target recognition; Sonar imagery | Issue Date: | 2023 | Journal/Edited collection: | 7th Underwater Acoustics Conference and Exhibition, UACE2023 | Start page: | 353 | End page: | 362 | Type: | Artikel/Aufsatz | Institution: | Hochschule Bremen | Faculty: | Hochschule Bremen - Fakultät 4: Elektrotechnik und Informatik |
Appears in Collections: | Bibliographie HS Bremen |
Items in Media are protected by copyright, with all rights reserved, unless otherwise indicated.