Citation link:
Publisher DOI: https://doi.org/10.1121/2.0001470
https://media.suub.uni-bremen.de/handle/elib/6580
Publisher DOI: https://doi.org/10.1121/2.0001470
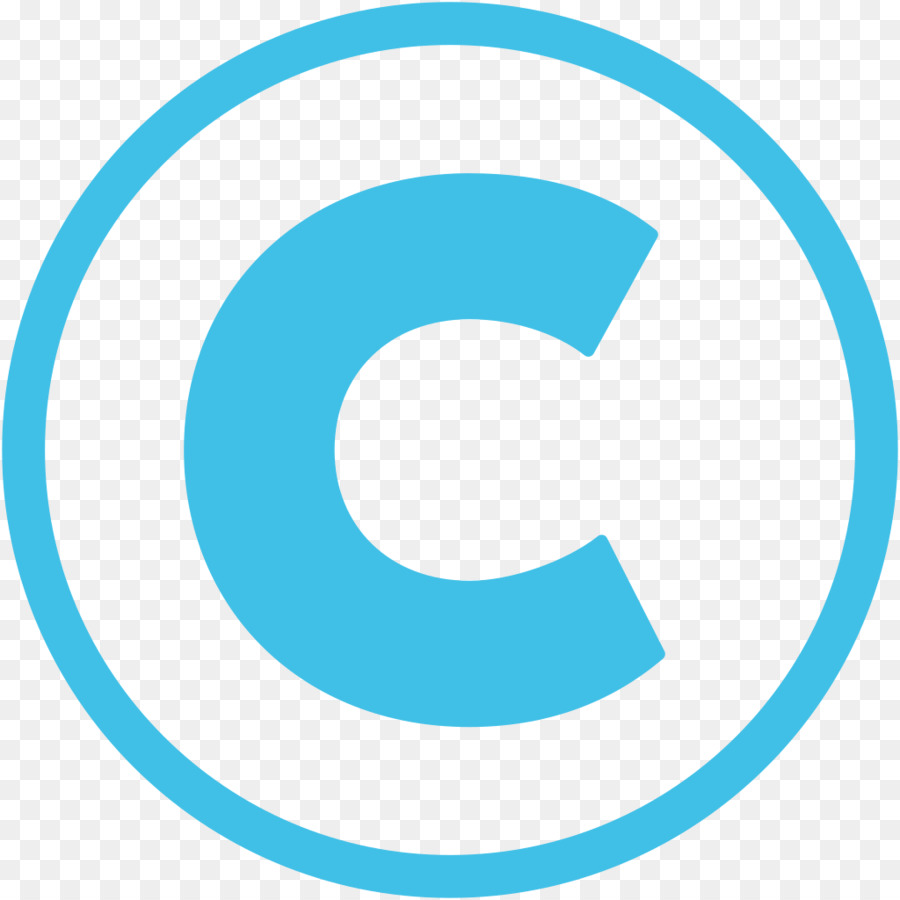
A study on modern deep learning detection algorithms for automatic target recognition in sidescan sonar images
Authors: | Steiniger, Yannik ![]() Groen, Johannes Stoppe, Jannis Ulrich ![]() Kraus, Dieter ![]() Meisen, Tobias ![]() |
Abstract: | State-of-the art deep learning models have shown remarkable performance on computer vision tasks like object classification or detection. These networks are typically trained on large-scale datasets of natural RGB images. However, sidescan sonar images are gray-scaled images representing acoustic intensities. The fundamental differences between camera and sonar as well as the images itself makes it necessary to investigate the transfer of results achieved on RGB images to the sonar imagery domain. Therefore, we compare the deep learning detection algorithm YOLOv2 with its updated version YOLOv3, both adopted for object detection in sidescan sonar images. In addition to this, a small convolutional neural network (CNN) is trained from scratch and used for detection. The experiments answer two questions: First, whether, as for general computer vision problems, transfer learning of large deep learning models is preferable over training of custom networks when dealing with limited sonar data. Secondly, whether improvements in the YOLO architecture, developed based on RGB images, lead to significant improvements on sonar data as well. Our results show that YOLOv3 indeed performs better than YOLOv2. Furthermore, YOLOv3 achieves a true positive rate of up to 98.2% and outperforms the small CNN. |
Issue Date: | 2021 | Publisher: | American Instut. of Physics | Journal/Edited collection: | Proceedings of Meetings on Acoustics | Series: | 6th Underwater Acoustics Conference and Exhibition | Issue: | 44 | Start page: | 070010 | Issue: | 44 | Type: | Artikel/Aufsatz | ISSN: | 1939-800X | Institution: | Hochschule Bremen | Faculty: | Hochschule Bremen - Fakultät 4: Elektrotechnik und Informatik |
Appears in Collections: | Bibliographie HS Bremen |
Items in Media are protected by copyright, with all rights reserved, unless otherwise indicated.