Citation link:
Publisher DOI: https://doi.org/10.3390/jmse9030239
https://media.suub.uni-bremen.de/handle/elib/6578
Publisher DOI: https://doi.org/10.3390/jmse9030239
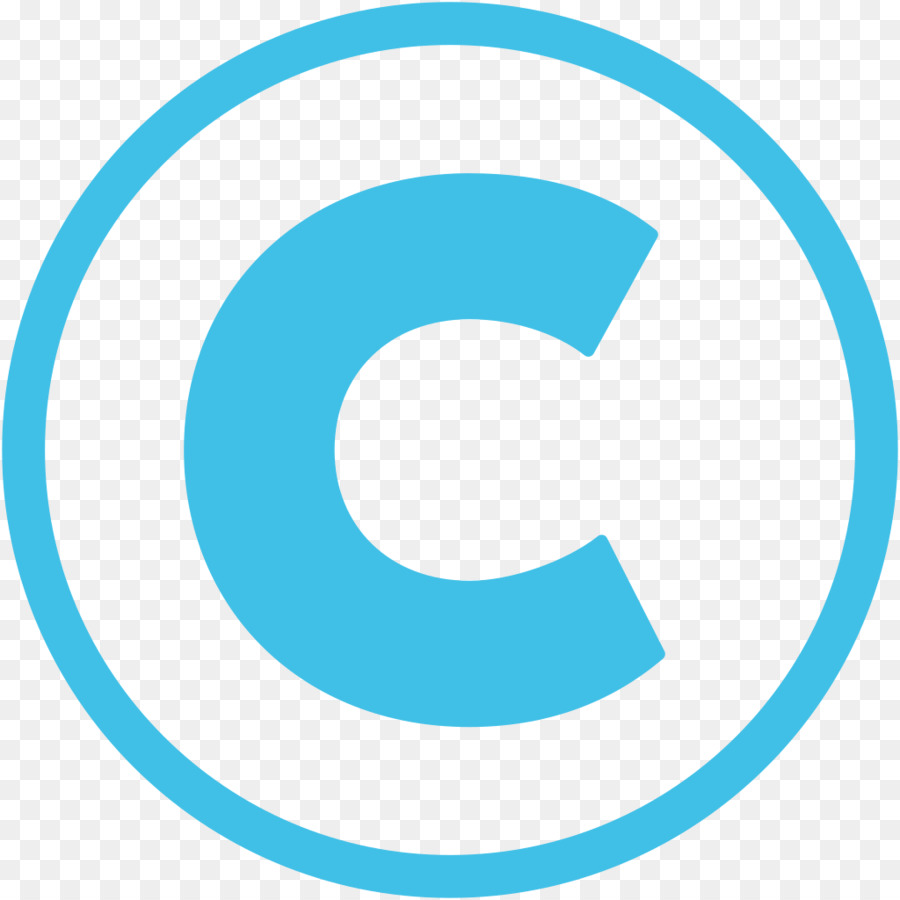
Generating Synthetic Sidescan Sonar Snippets Using Transfer-Learning in Generative Adversarial Networks
Authors: | Steiniger, Yannik ![]() Kraus, Dieter ![]() Meisen, Tobias ![]() |
Abstract: | The training of a deep learning model requires a large amount of data. In case of sidescan sonar images, the number of snippets from objects of interest is limited. Generative adversarial networks (GAN) have shown to be able to generate photo-realistic images. Hence, we use a GAN to augment a baseline sidescan image dataset with synthetic snippets. Although the training of a GAN with few data samples is likely to cause mode collapse, a combination of pre-training using simple simulated images and fine-tuning with real data reduces this problem. However, for sonar data, we show that this approach of transfer-learning a GAN is sensitive to the pre-training step, meaning that the vanishing of the gradients of the GAN’s discriminator becomes a critical problem. Here, we demonstrate how to overcome this problem, and thus how to apply transfer-learning to GANs for generating synthetic sidescan snippets in a more robust way. Additionally, in order to further investigate the GAN’s ability to augment a sidescan image dataset, the generated images are analyzed in the image and the frequency domain. The work helps other researchers in the field of sonar image processing to augment their dataset with additional synthetic samples. |
Keywords: | deep learning; generative adversarial networks; transfer-learning; sidescan sonar; synthetic sonar images | Issue Date: | 2021 | Publisher: | MDPI | Journal/Edited collection: | Journal of Marine Science and Engineering | Issue: | 3 | Start page: | 239 | Volume: | 9 | Type: | Artikel/Aufsatz | ISSN: | 2077-1312 | Institution: | Hochschule Bremen | Faculty: | Hochschule Bremen - Fakultät 4: Elektrotechnik und Informatik |
Appears in Collections: | Bibliographie HS Bremen |
Items in Media are protected by copyright, with all rights reserved, unless otherwise indicated.