Citation link:
https://media.suub.uni-bremen.de/handle/elib/6416
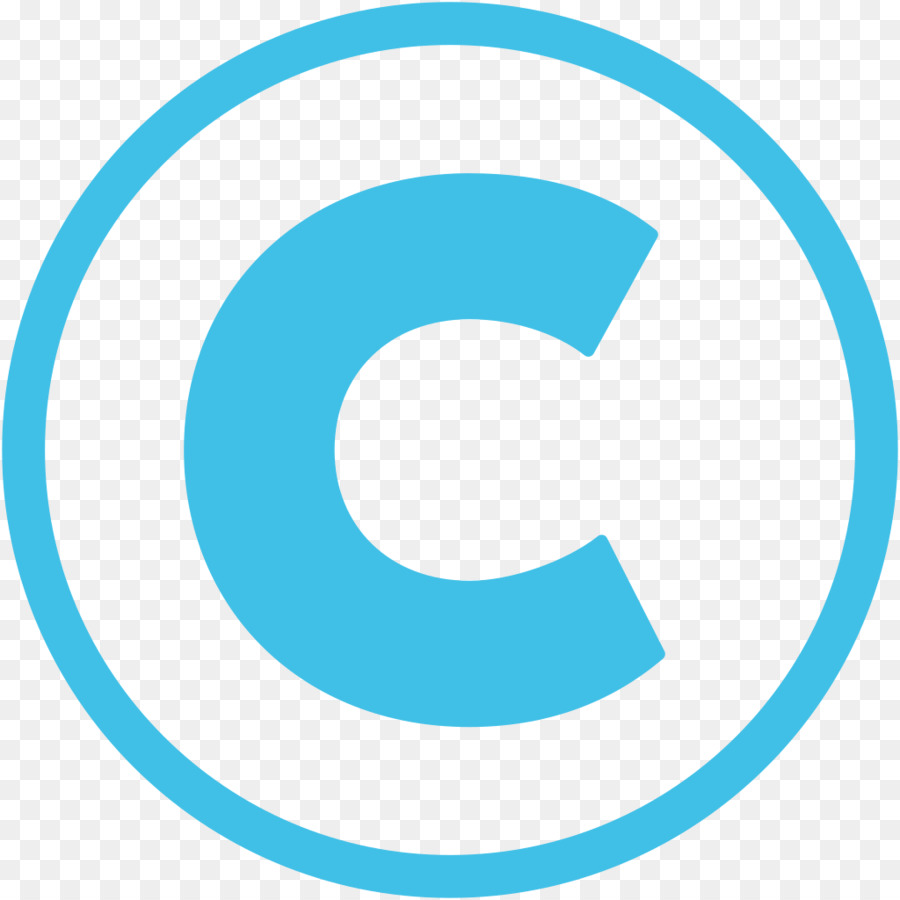
A Dimensionality Reduction Method for Finding Least Favorable Priors with a Focus on Bregman Divergence
Authors: | Goldenbaum, Mario ![]() Dytso, Alex ![]() Poor, H. Vincent ![]() Shamai Shitz, Shlomo ![]() |
Abstract: | A common way of characterizing minimax estimators in point estimation is by moving the problem into the Bayesian estimation domain and finding a least favorable prior distribution. The Bayesian estimator induced by a least favorable prior, under mild conditions, is then known to be minimax. However, finding least favorable distributions can be challenging due to inherent optimization over the space of probability distributions, which is infinite-dimensional. This paper develops a dimensionality reduction method that allows us to move the optimization to a finite-dimensional setting with an explicit bound on the dimension. The benefit of this dimensionality reduction is that it permits the use of popular algorithms such as projected gradient ascent to find least favorable priors. Throughout the paper, in order to make progress on the problem, we restrict ourselves to Bayesian risks induced by a relatively large class of loss functions, namely Bregman divergences. |
Keywords: | Bregman Divergence | Issue Date: | 2022 | Publisher: | PMLR | Journal/Edited collection: | Proceedings of Machine Learning Research | Start page: | 8080 | End page: | 8094 | Volume: | 151 | Type: | Artikel/Aufsatz | ISSN: | 2640-3498 | Institution: | Hochschule Bremen | Faculty: | Hochschule Bremen - Fakultät 4: Elektrotechnik und Informatik |
Appears in Collections: | Bibliographie HS Bremen |
Items in Media are protected by copyright, with all rights reserved, unless otherwise indicated.