Citation link:
Publisher DOI: https://doi.org/10.1109/OCEANS47191.2022.9977255
https://media.suub.uni-bremen.de/handle/elib/6582
Publisher DOI: https://doi.org/10.1109/OCEANS47191.2022.9977255
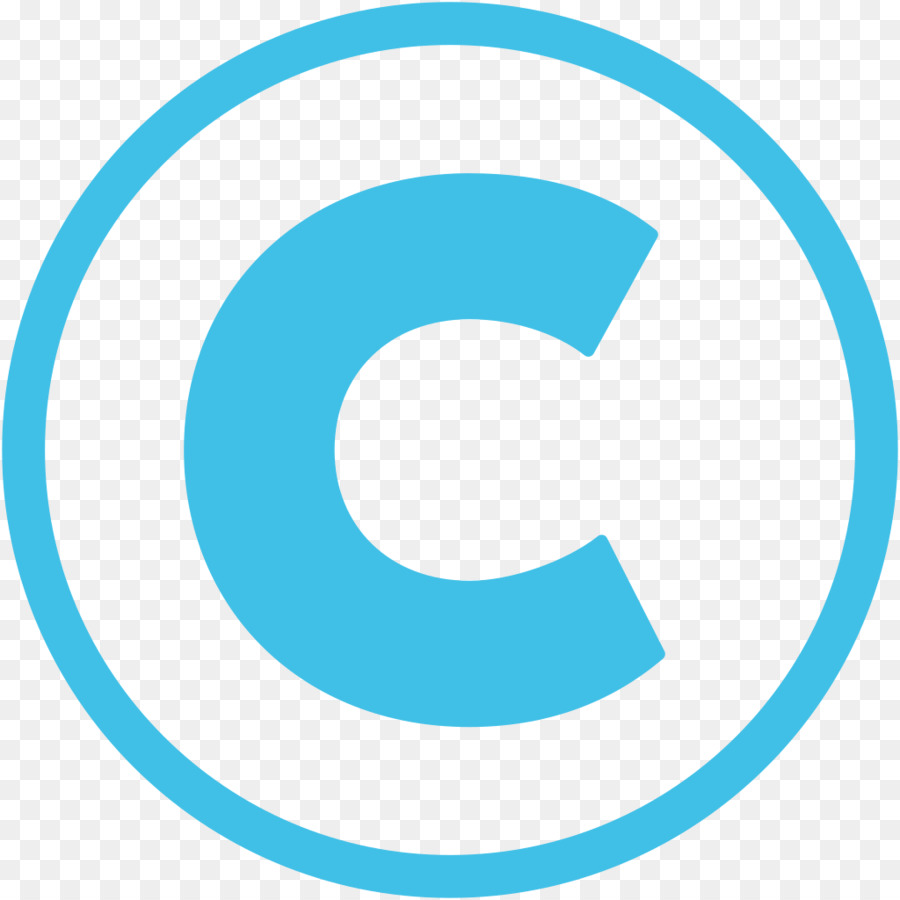
Investigating the training of convolutional neural networks with limited sidescan sonar image datasets
Authors: | Steiniger, Yannik ![]() Stoppe, Jannis Ulrich ![]() Kraus, Dieter ![]() Meisen, Tobias ![]() |
Abstract: | For the automatic analysis of sonar images, deep learning methods, like convolutional neural networks (CNN), outperform traditional approaches. However, the training of a CNN requires a sufficient dataset, which is difficult to obtain. Thus, ways to improve the training of CNNs with limited data are necessary to reach a reasonable performance of the networks under such circumstances. In our work we consider an extreme case of having less than 20 samples per class to train a CNN for classification. We train two custom CNNs with various depths from scratch, as well as the even deeper VGG-16 network, which is a commonly used architecture for sonar imagery. Furthermore, the benefits of several augmentation methods in the sonar imagery context are investigated. In addition, we study how the image complexity can be reduced so that a CNN can learn more efficiently with the limited training data. Besides, we evaluate if we can feed additional features to the CNN for a further improvement. Our best model is a VGG-16 which is trained using flipping, cropping and addition of Gaussian noise for data augmentation. The complexity of the input images is reduced using tight bounding boxes for snippet extraction, which remove the object size information. Adding this size information as an explicit feature to the CNN increases the performance and results in a balanced accuracy of 77.2%. |
Keywords: | deep learning; sidescan sonar; Sonar imagery; computer vision | Issue Date: | 2022 | Publisher: | IEEE | Journal/Edited collection: | Oceans 2022 | Start page: | 1 | End page: | 6 | Type: | Artikel/Aufsatz | ISBN: | 978-1-6654-6809-1 | ISSN: | 978-1-6654-1821-8 | Institution: | Hochschule Bremen | Faculty: | Hochschule Bremen - Fakultät 4: Elektrotechnik und Informatik |
Appears in Collections: | Bibliographie HS Bremen |
Items in Media are protected by copyright, with all rights reserved, unless otherwise indicated.